"Intervention" is required when otherwise intelligent people share Russian-style disinformation, e.g., re: DONALD JOHN TRUMP and his works and pomps. I was disappointed to watch faux Fox News video of the former Miami U.S. Attorney, GUY ALAN LEWIS, predicting TRUMP's acquittal a week ago, and then perseverating and ululating about DJT's conviction of 34 felony counts of causing false business records to be created. As JFK said, "Sometimes party loyalty demands too much." Fun fact: GUY ALAN LEWIS was my law school classmate; he and I took Constitutional Law with Professor Claude Coffman in Memphis, both hearing grades of "A." From SCIENCE Magazine, published by the American Association for the Advancement of Science:
A broader view of misinformation reveals potential for intervention
Misinformation is viewed as a threat to science, public health, and democracies worldwide (1). Experts define misinformation as content that is false or misleading, such that it contains some facts but is otherwise manipulative (2, 3). Yet, the importance of this distinction has remained unquantified. On pages 978 and 979 of this issue, Allen et al. (4) and Baribi-Bartov et al. (5), respectively, report on the impact of misinformation on social media. Allen et al. find that Facebook content not flagged as misinformation but still expressing misleading views on vaccinations had a much bigger effect on vaccination intentions compared with outright falsehoods because of its greater reach. Baribi-Bartov et al. investigate who is responsible for spreading misinformation about voting on X (previously Twitter), identifying highly networked citizens (“supersharers”) who supply about a quarter of the fake news received by their followers. These findings highlight new ways to intervene in misinformation propagation.
The global spread of misinformation can have deleterious consequences for society, including election denial and vaccine hesitancy (1, 2, 6). Yet, some scholars have criticized such claims, noting that quantitative evidence of the causal impact of misinformation—and those who spread it—is often lacking and limited to self-reported data or laboratory conditions that may not generalize to the real world (7). Although this is a valid concern, it would neither be ethical nor feasible to randomly assign half the population to misinformation about vaccines or elections to determine whether that changes vaccine uptake or election outcomes. The tobacco industry weaponized the lack of direct causal evidence for the impact of smoking on lung cancer by manipulating public opinion and casting doubt on the negative health consequences of smoking, even though plenty of other good evidence was available to inform action (8). It is therefore important to have realistic expectations for standards of evidence.
Allen et al. combine high-powered controlled experiments with machine learning and social media data on roughly 2.7 billion vaccine-related URL views on Facebook during the rollout of the first COVID-19 vaccine at the start of 2021. Contrary to claims that misinformation does not affect choices (7), the causal estimates reported by Allen et al. suggest that exposure to a single piece of vaccine misinformation reduced vaccination intentions by ∼1.5 percentage points. Particularly noteworthy is that when predicting how big an impact misinformation exerts on vaccination intentions, what mattered most was not the veracity of the article but rather the extent to which the article claimed that vaccines were harmful to people’s health. A key example of such true-but-misleading content is the following headline from the Chicago Tribune: “A ‘healthy’ doctor died two weeks after getting a COVID-19 vaccine; CDC is investigating why.” This headline is misleading because the framing falsely implies causation where there is only correlation (i.e., there was no evidence that the vaccine had anything to do with the death of the doctor). Nonetheless, this headline was viewed by nearly 55 million people on Facebook, which is more than six times the exposure of all factchecked misinformation combined.
In the final step, Allen et al. assign weight to the treatment (i.e., persuasion) effect (estimated with a machine learning classifier) by looking at the number of exposures for each URL on the platform. To accomplish this, they obtained 13,206 URLs about COVID-19 vaccines on Facebook in 2021. By differentiating URLs flagged as misinformation by fact-checkers from those not flagged but still hesitancy inducing, the authors compared the impact of false versus misleading information. About 98% of the total 500 million URL views came from misleading content rather than content that was fact-checked as false. When taking the product of exposure and treatment impact, the authors estimate that vaccineskeptical content not flagged by fact-checkers reduced vaccination intentions by 2.8 percentage points relative to just 0.05 percentage points for blatant falsehoods. In other words, the negative impact of misleading content on the intention to receive a COVID-19 vaccine was 46 times that of content flagged as misinformation. Considering the 233 million Facebook users, preventing exposure to this type of content would have resulted in at least 3 million more vaccinated Americans.
Misleading information makes up a much larger proportion of misinformation than fact-checked fake news and has a higher impact on society because more people get exposed to it, potentially through supersharers. Interventions to reduce the public’s susceptibility to misleading techniques and the reach of supersharers could lower the impact of misinformation. Darker nodes represent fake and misleading news supersharers, and lighter nodes represent members of the public exposed to fake or misleading news.
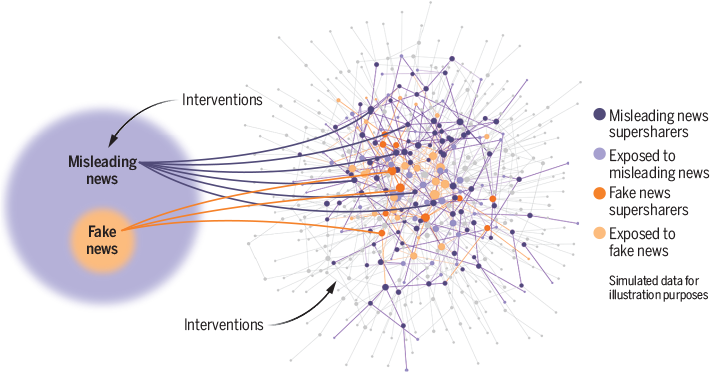
One important caveat is that intentions do not always translate into behavior, but the above predictions account for this by assuming that the impact of misinformation on actual vaccination rates is about 60% of the effect on the intention to get vaccinated, which is consistent with estimates of the intention-behavior gap (9). Moreover, these estimates do not include visual misinformation or content shared on other social media platforms and thus likely represent a lower bound for the true impact of misinformation.
The findings from Allen et al. do not address who is spreading most of the misinformation, which is important to understand because supersharers undermine democratic representation by flooding the online space with false information. Baribi-Bartov et al. profile 2107 registered US voters (0.3% of the total panel of 664,391 voters matched to active Twitter users) who were responsible for 80% of misinformation shared on X during the 2020 US presidential election. One of the key insights from their study is that these supersharers receive more engagement than regular users and are highly connected, ranking in the 86th percentile of network influence. The authors also mapped the content that users in the panel are exposed to based on their following, which revealed that supersharers supply nearly 25% of the misinformation available to their followers. In terms of their profile, supersharers had a higher likelihood of being (female) Republican, white, and older (average of 58.2 years of age). Although consistent with other preliminary work in the context of US elections (10), this profile is unlikely to be universal and thus may not generalize to other contexts.
An important difference between the two studies is that whereas Allen et al. draw attention to misleading news from mainstream sources, Baribi-Bartov et al. focus on the narrower class of fake news websites. An important area for future research would therefore be to consider supersharers of misleading information, regardless of the source (see the figure). For example, the Chicago Tribune headline was published by a mainstream high-quality source but was heavily pushed by antivaccination groups on Facebook (11), who could be supersharers and responsible for a substantial portion of the traffic. These results also broadly align with other recent work that identified 52 US physicians on social media as supersharers of COVID-19 misinformation (12). Baribi-Bartov et al. do not speculate on motives, but related work finds that supersharers are diverse, including political pundits, media personalities, contrarians, and antivaxxers with personal, financial, and political motives for spreading untrustworthy content (10).
Although research studies often define misinformation as a set of stimuli that have been fact-checked as true or false (3, 13), the most persuasive forms of misinformation are likely misleading claims published by mainstream sources subsequently pushed by well-networked supersharers. This has considerable ramifications for designing interventions to counter misinformation, most of which are focused on blatant falsehoods (13). One option is to suppress, flag, or moderate content, given that supersharers represent a tiny fraction of the population but have the potential to cause outsized harm. The downside is that such an approach often raises concerns about free speech. Another option is psychological inoculation or prebunking (14), which specifically targets manipulation techniques often present in true-but-misleading content, such as polarization, fearmongering, and false dichotomies. Inoculation preemptively exposes users to a weakened dose of the tactic to prevent full-scale manipulation and has been scaled on social media to millions of users. For example, short videos alerting users to false dichotomies and decontextualization have been implemented as You-Tube advertisements (15) before people are exposed to potential misinformation. Social media companies could identify supersharers and inoculate them and their networks against such manipulation techniques.
Department of Psychology, Downing Site, University of Cambridge, Cambridge, UK. Email: sander.vanderlinden@psychol.cam.ac.uk
REFERENCES AND NOTES
ACKNOWLEDGMENTS
The authors receive funding from the Bill and Melinda Gates Foundation, the European Commission, and Google.
10.1126/science.adp9117